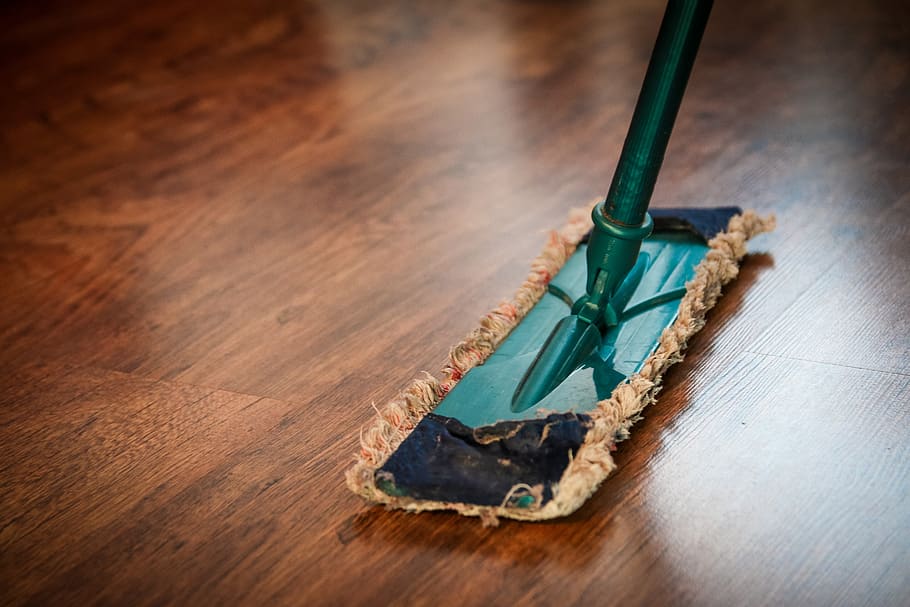
One of the most annoying parts of data work is dealing with inconsistent entities: names of the same person spelled differently. Company names that rebranded, merged, or have varying suffixes like “Ltd.” and “Limited”.
Standardizing data for accurate analysis can take days, sometime weeks, even with powerful tools like OpenRefine and Dedupe, which were made for this purpose.
With generative AI becoming so powerful, can it be used to clean up messy datasets with less human intervention?
I put ChatGPT to the test on several data cleaning tasks.
TL;DR: It shows great potential, but like with anything critical, it’s not quite ready to be trusted 100%, at least not on the current models (I’m using the free ChatGPT version with the older GPT-3.5 model). Accuracy might also improve with better-crafted prompts.
Standardizing company names
I gave ChatGPT a list of company names that I know have either changed or been acquired. I wanted to see how well it knows the more recent name and the parent company.
Prompt:
Today we are going to standardize company names. Create a table with the company names provided and another column with that company’s most recent name. Add a third column with the company’s parent company. Only provide the company name without legal abbreviations like “Ltd.”, “Corp.”, “LLC” or other suffixes.
Bell Canada
Rogers Media
Koodo Mobile
Grand Trunk Western Railroad
Molson Brewery
General Dynamics Mission Systems–Canada
Google Canada
Facebook Ltd.
Nintendo of America
Rio Tinto
Teck Cominco
CP Rail
Encana Corp.
Canadian Natural
L3 Technologies
Storm Resources
Ubisoft Montreal
AWS web services
RIM
Abitibi Consolidated
Future Shop
Videotron Ltd.
RBC Secutiries
Zellers
Grand River Railway
Response:
Original Name | Most Recent Name | Parent Company |
---|---|---|
Bell Canada | Bell Canada | BCE Inc. |
Rogers Media | Rogers Sports & Media | Rogers Communications |
Koodo Mobile | Koodo Mobile | Telus Communications |
Grand Trunk Western Railroad | Canadian National Railway | Canadian National Railway |
Molson Brewery | Molson Coors Brewing Company | Molson Coors |
General Dynamics Mission Systems–Canada | General Dynamics Mission Systems Canada | General Dynamics |
Google Canada | Google Canada | Alphabet Inc. |
Facebook Ltd. | Facebook Inc. | |
Nintendo of America | Nintendo of America | Nintendo Co., Ltd. |
Rio Tinto | Rio Tinto | Rio Tinto Group |
Teck Cominco | Teck Resources | Teck Resources Limited |
CP Rail | Canadian Pacific Railway | Canadian Pacific Railway |
Encana Corp. | Ovintiv Inc. | Ovintiv Inc. |
Canadian Natural | Canadian Natural Resources Limited | Canadian Natural Resources |
L3 Technologies | L3Harris Technologies | L3Harris Technologies |
Storm Resources | ARC Resources Ltd. | ARC Resources Ltd. |
Ubisoft Montreal | Ubisoft Montreal | Ubisoft Entertainment |
AWS web services | Amazon Web Services | Amazon.com, Inc. |
RIM | BlackBerry Limited | BlackBerry Limited |
Abitibi Consolidated | Resolute Forest Products | Resolute Forest Products |
Future Shop | Best Buy Canada | Best Buy Co., Inc. |
Videotron Ltd. | Videotron | Quebecor Inc. |
RBC Securities | RBC Capital Markets | Royal Bank of Canada |
Zellers | Target Canada | Target Corporation |
Grand River Railway | Canadian Pacific Railway | Canadian Pacific Railway |
Comments:
For the most part, it was spot on. It knows that companies like Zellers and Future Shop were acquired and it knows their parent companies. It also knows that Encana rebranded to Ovintiv.
BUT it got one thing very wrong. Storm Resources was acquired by Canadian Natural Resources, not ARC. It also didn’t put Meta as Facebook’s parent company. Although minor, it didn’t follow the instructions to exclude legal suffixes like “Ltd.”
Standardizing people names
I gave ChatGPT a list of names. Some of them are correct, others have minor typos, middle initials, and nicknames. I asked it to standardize the names to the same form.
Prompt:
Below is a list of names. Some of those names are repeated but have spelling mistakes, nicknames and middle initials. Create a table with these names and add a column with a standardized version of the name: fix typos, remove middle initials, and convert nicknames to proper first names.
Navdeep Singh Bains
Jason Kenney
Mary Ng
Thomas McKenzie
Edward Gallagher
Harjit Sajjan
Mary Ngg
Helen Rosenthal
Sam Ho
Jason T. Kenney
Helen A Rosenthal
Tom McKenzie
Navdeep Bains
Edward Gallager
Harjitt Sajan
Helen Rosental
Samuel Ho
Francois Pelletier
Jean Marc Fournier
Marisa Lopez
Jean-Marc Fournier
François Pelletier
Thomas Mackenzie
Marissa Lopez
Response: (fixed names in bold)
Original Name | Standardized Name |
---|---|
Navdeep Singh Bains | Navdeep Bains |
Jason Kenney | Jason Kenney |
Mary Ng | Mary Ng |
Thomas McKenzie | Thomas McKenzie |
Edward Gallagher | Edward Gallagher |
Harjit Sajjan | Harjit Sajjan |
Mary Ngg | Mary Ng |
Helen Rosenthal | Helen Rosenthal |
Sam Ho | Samuel Ho |
Jason T. Kenney | Jason Kenney |
Helen A Rosenthal | Helen Rosenthal |
Tom McKenzie | Thomas McKenzie |
Edward Gallager | Edward Gallagher |
Harjitt Sajan | Harjit Sajjan |
Helen Rosental | Helen Rosenthal |
Samuel Ho | Samuel Ho |
Francois Pelletier | François Pelletier |
Jean Marc Fournier | Jean-Marc Fournier |
Marisa Lopez | Marisa Lopez |
Jean-Marc Fournier | Jean-Marc Fournier |
François Pelletier | François Pelletier |
Thomas Mackenzie | Thomas McKenzie |
Marissa Lopez | Marisa Lopez |
Comment:
For this small sample, ChatGPT did exactly what was asked of it. Typos were fixed, initials stripped, and nicknames converted to proper first names. French compound names were hyphenated correctly.
However, there’s a danger inherent in standardizing names like this. Helen Rosenthal and Helen A Rosenthal could indeed be different people. There could certainly be a Marisa Lopez and an unrelated Marissa. Although this could be a big time saver, it has to be done cautiously and respectful of the data’s context.
Enriching data: assigning NAICS codes to company names
ChatGPT is not only useful at fixing data, but it can also enrich data by adding new fields. This is called feature engineering in data science and it can be laborious if two datasets don’t match perfectly.
Suppose I have a list of companies and I want to know their industrial sector. The NAICS industry classification system is a great standardized list of company types, but there are no readily-available resources that map companies to sector codes. ChatGPT makes this trivial.
Prompt:
Now we’re going to classify companies by industrial sector. Below is a list of companies. Create a table with the company name and add a column with its three-digit NAICS code. Add a third column with the NAICS subsector name.
Bell Canada
Rogers Media
Molson Coors
General Dynamics
Google Canada
Meta Ltd.
Rio Tinto
CP Rail
Canadian Natural Resources
L3Harris
Ubisoft
AWS
Scotiabank
CGI Inc.
Hewlett Packard
Ikea
Raytheon
Broil King
Volkswagen
Response:
Company Name | NAICS Code | NAICS Subsector Name |
---|---|---|
Bell Canada | 517 | Telecommunications |
Rogers Media | 515 | Broadcasting (except Internet) |
Molson Coors | 312 | Breweries |
General Dynamics | 336 | Aerospace Product and Parts Manufacturing |
Google Canada | 518 | Data Processing, Hosting, and Related Services |
Meta Ltd. | 518 | Data Processing, Hosting, and Related Services |
Rio Tinto | 212 | Mining (except Oil and Gas) |
CP Rail | 482 | Rail Transportation |
Canadian Natural Resources | 211 | Oil and Gas Extraction |
L3Harris | 334 | Computer and Electronic Product Manufacturing |
Ubisoft | 511 | Publishing Industries (except Internet) |
AWS | 518 | Data Processing, Hosting, and Related Services |
Scotiabank | 522 | Credit Intermediation and Related Activities |
CGI Inc. | 541 | Professional, Scientific, and Technical Services |
Hewlett Packard | 334 | Computer and Electronic Product Manufacturing |
Ikea | 442 | Furniture and Home Furnishing Merchant Wholesalers |
Raytheon | 334 | Computer and Electronic Product Manufacturing |
Broil King | 333 | Machinery Manufacturing |
Volkswagen | 336 | Aerospace Product and Parts Manufacturing |
Comment:
ChatGPT got the NAICS code for every company right. The subsector names are iffy, but this shows the importance of a good prompt. I asked ChatGPT to give me the subsector name, but didn’t specify the granularity. So sometimes it gave me a general three-digit descriptor, but sometimes it went deeper down the hierarchy.
Take Molson Coors. The 312 NAICS code is “Beverage and tobacco product manufacturing”, but ChatGPT chose the more granular five-digit subsector of “Breweries” for the descriptor.
Likewise, Volkswagen is certainly 336 (Transportation equipment manufacturing), and it’s primarily an auto manufacturer, although it has entered the electric vertical-takeoff-and-landing (eVTOL) market. Maybe this is why it got labelled as an aerospace manufacturer?
Also, large conglomerate can be tricky to categorize. A huge defense contractor like L3Harris, which makes military drones and satellite communications systems, was given the general “Computer and Electronic Product Manufacturing” label.
Conclusion
Processing data with ChatGPT shows a lot of promise, but it’s still not ready to be fully automated and trusted. Maybe future, better models will solve these problems, or maybe it’s all about crafting better prompts.
Also, these were small testing samples. Piping large datasets through the AI can be tricky, require the use of coding APIs, and can be a lot harder to validate.
Have you used LLMs to clean and enrich data? Share your experiences below.
Tried again with GPT 4 – I’m struggling with latest model at the moment with this task
Here is a table classifying the listed companies by their respective three-digit NAICS codes and corresponding subsector names:
Company Name NAICS Code NAICS Subsector
Bell Canada 517 Telecommunications
Rogers Media 517 Telecommunications
Molson Coors 312 Beverage and Tobacco Product Manufacturing
General Dynamics 336 Transportation Equipment Manufacturing
Google Canada 518 Data Processing, Hosting, and Related Services
Meta Ltd. 519 Other Information Services
Rio Tinto 212 Mining (except Oil and Gas)
CP Rail 482 Rail Transportation
Canadian Natural Resources 211 Oil and Gas Extraction
L3Harris 334 Computer and Electronic Product Manufacturing
Ubisoft 511 Publishing Industries (except Internet)
AWS 518 Data Processing, Hosting, and Related Services
Scotiabank 522 Finance and Insurance
CGI Inc. 541 Professional, Scientific, and Technical Services
Hewlett Packard 334 Computer and Electronic Product Manufacturing
Ikea 442 Furniture and Home Furnishings Stores
Raytheon 336 Transportation Equipment Manufacturing
Broil King 335 Electrical Equipment, Appliance, and Component Manufacturing
Volkswagen 336 Transportation Equipment Manufacturing
This looks pretty good to me. What’s the issue you see? I also had better results with GPT-4, making sure to specify in the prompt the granularity of the NAICS code and its descriptor.